Yet another tech startup wants to topple Nvidia with ‘orders of magnitude’ better energy efficiency; Sagence AI bets on analog in-memory compute to deliver 666K tokens/s on Llama2-70B

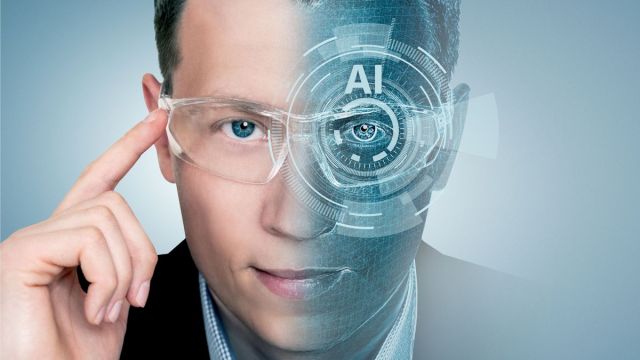
- Sagence brings analog in-memory compute to redefine AI inference
- Ten times lower power and 20 times lower costs
- Also offers integration with PyTorch and TensorFlow
Sagence AI has introduced an advanced analog in-memory compute architecture designed to address issues of power, cost, and scalability in AI inference.
Using an analog-based approach, the architecture offers improvements in energy efficiency and cost-effectiveness while delivering performance comparable to existing high-end GPU and CPU systems.
This bold step positions Sagence AI as a potential disruptor in a market dominated by Nvidia.
Efficiency and performance
The Sagence architecture offers benefits when processing large language models like Llama2-70B. When normalized to 666,000 tokens per second, Sagence’s technology delivers its results with 10 times lower power consumption, 20 times lower costs, and 20 times smaller rack space compared to leading GPU-based solutions.
This design prioritizes the demands of inference over training, reflecting the shift in AI compute focus within data centers. With its efficiency and affordability, Sagence offers a solution to the growing challenge of ensuring return on investment (ROI) as AI applications expand to large-scale deployment.
At the heart of Sagence’s innovation is its analog in-memory computing technology, which merges storage and computation within memory cells. By eliminating the need for separate storage and scheduled multiply-accumulate circuits, this approach simplifies chip designs, reduces costs, and improves power efficiency.
Sagence also employs deep subthreshold computing in multi-level memory cells – an industry-first innovation – to achieve the efficiency gains required for scalable AI inference.
Sign up to the TechRadar Pro newsletter to get all the top news, opinion, features and guidance your business needs to succeed!
Traditional CPU and GPU-based systems rely on complex dynamic scheduling, which increases hardware demands, inefficiencies, and power consumption. Sagence’s statically scheduled architecture simplifies these processes, mirroring biological neural networks.
The system is also designed to integrate with existing AI development frameworks like PyTorch, ONNX, and TensorFlow. Once trained neural networks are imported, Sagence’s architecture negates the need for further GPU-based processing, simplifying deployment and reducing costs.
“A fundamental advancement in AI inference hardware is vital to the future of AI. Use of large language models (LLMs) and Generative AI drives demand for rapid and massive change at the nucleus of computing, requiring an unprecedented combination of highest performance at lowest power and economics that match costs to the value created,” said Vishal Sarin, CEO & Founder, Sagence AI.
“The legacy computing devices today that are capable of extreme high-performance AI inferencing cost too much to be economically viable and consume too much energy to be environmentally sustainable. Our mission is to break those performance and economic limitations in an environmentally responsible way,” Sarin added.
Via IEEE Spectrum
You may also like
Sagence brings analog in-memory compute to redefine AI inference Ten times lower power and 20 times lower costs Also offers integration with PyTorch and TensorFlow Sagence AI has introduced an advanced analog in-memory compute architecture designed to address issues of power, cost, and scalability in AI inference. Using an analog-based…
Recent Posts
- Grok blocked results saying Musk and Trump “spread misinformation”
- A GPU or a CPU with 4TB HBM-class memory? Nope, you’re not dreaming, Sandisk is working on such a monstrous product
- The Space Force shares a photo of Earth taken by the X-37B space plane
- Elon Musk claims federal employees have 48 hours to explain recent work or resign
- xAI could sign a $5 billion deal with Dell for thousands of servers with Nvidia’s GB200 Blackwell AI GPU accelerators
Archives
- February 2025
- January 2025
- December 2024
- November 2024
- October 2024
- September 2024
- August 2024
- July 2024
- June 2024
- May 2024
- April 2024
- March 2024
- February 2024
- January 2024
- December 2023
- November 2023
- October 2023
- September 2023
- August 2023
- July 2023
- June 2023
- May 2023
- April 2023
- March 2023
- February 2023
- January 2023
- December 2022
- November 2022
- October 2022
- September 2022
- August 2022
- July 2022
- June 2022
- May 2022
- April 2022
- March 2022
- February 2022
- January 2022
- December 2021
- November 2021
- October 2021
- September 2021
- August 2021
- July 2021
- June 2021
- May 2021
- April 2021
- March 2021
- February 2021
- January 2021
- December 2020
- November 2020
- October 2020
- September 2020
- August 2020
- July 2020
- June 2020
- May 2020
- April 2020
- March 2020
- February 2020
- January 2020
- December 2019
- November 2019
- September 2018
- October 2017
- December 2011
- August 2010