Intel’s former CEO puts money into a little-known hardware startup that wants to make Nvidia obsolete
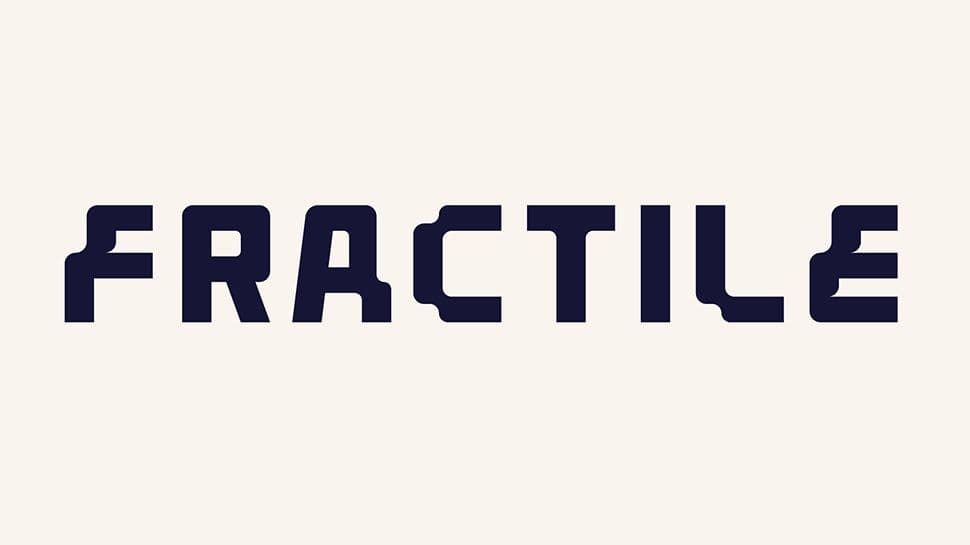
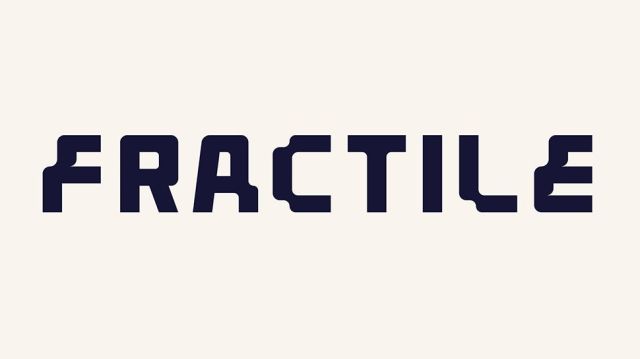
- UK-based Fractile is backed by NATO and wants to build faster and cheaper in-memory AI compute
- Nvidia’s bruteforce GPU approach consumes too much power and is held back by memory
- Fractile’s numbers focused on a cluster of H100 GPU comparison, not the mainstream H200
Nvidia sits comfortably at the top of the AI hardware food chain, dominating the market with its high-performance GPUs and CUDA software stack, which have quickly become the default tools for training and running large AI models – but that dominance comes at a cost – namely, a growing target on its back.
Hyperscalers like Amazon, Google, Microsoft and Meta are pouring resources into developing their own custom silicon in an effort to reduce their dependence on Nvidia’s chips and cut costs. At the same time, a wave of AI hardware startups is trying to capitalize on rising demand for specialized accelerators, hoping to offer more efficient or affordable alternatives and, ultimately, to displace Nvidia.
You may not have heard of UK-based Fractile yet, but the startup, which claims its revolutionary approach to computing can run the world’s largest language models 100x faster and at 1/10th the cost of existing systems, has some pretty noteworthy backers, including NATO and the former CEO of Intel, Pat Gelsinger.
Removing every bottleneck
“We are building the hardware that will remove every bottleneck to the fastest possible inference of the largest transformer networks,” Fractile says.
“This means the biggest LLMs in the world running faster than you can read, and a universe of completely new capabilities and possibilities for how we work that will be unlocked by near-instant inference of models with superhuman intelligence.”
It’s worth pointing out, before you get too excited, that Fractile’s performance numbers are based on comparisons with clusters of Nvidia H100 GPUs using 8-bit quantization and TensorRT-LLM, running Llama 2 70B – not the newer H200 chips.
In a LinkedIn posting, Gelsinger, who recently joined VC firm Playground Global as a General Partner, wrote, “Inference of frontier AI models is bottlenecked by hardware. Even before test-time compute scaling, cost and latency were huge challenges for large scale LLM deployments… To achieve our aspirations for AI, we will need radically faster, cheaper and much lower power inference.”
Sign up to the TechRadar Pro newsletter to get all the top news, opinion, features and guidance your business needs to succeed!
“I’m pleased to share that I’ve recently invested in Fractile, a UK-founded AI hardware company who are pursuing a path that’s radical enough to offer such a leap,” he then revealed.
“Their in-memory compute approach to inference acceleration jointly tackles the two bottlenecks to scaling inference, overcoming both the memory bottleneck that holds back today’s GPUs, while decimating power consumption, the single biggest physical constraint we face over the next decade in scaling up data center capacity. In fact, some of the ideas I was exploring in my graduate work at Stanford University will now come to mainstream AI computing!”
You might also like
UK-based Fractile is backed by NATO and wants to build faster and cheaper in-memory AI compute Nvidia’s bruteforce GPU approach consumes too much power and is held back by memory Fractile’s numbers focused on a cluster of H100 GPU comparison, not the mainstream H200 Nvidia sits comfortably at the top…
Recent Posts
- Razer pauses direct laptop sales in the US as new tariffs loom
- Intel’s former CEO puts money into a little-known hardware startup that wants to make Nvidia obsolete
- CrushFTP vulnerability exploited in the wild, added to CISA KEV database
- Hades II will launch on Switch 2 and Switch before PlayStation and Xbox
- YouTube says Nope! to age-gating Balatro videos
Archives
- April 2025
- March 2025
- February 2025
- January 2025
- December 2024
- November 2024
- October 2024
- September 2024
- August 2024
- July 2024
- June 2024
- May 2024
- April 2024
- March 2024
- February 2024
- January 2024
- December 2023
- November 2023
- October 2023
- September 2023
- August 2023
- July 2023
- June 2023
- May 2023
- April 2023
- March 2023
- February 2023
- January 2023
- December 2022
- November 2022
- October 2022
- September 2022
- August 2022
- July 2022
- June 2022
- May 2022
- April 2022
- March 2022
- February 2022
- January 2022
- December 2021
- November 2021
- October 2021
- September 2021
- August 2021
- July 2021
- June 2021
- May 2021
- April 2021
- March 2021
- February 2021
- January 2021
- December 2020
- November 2020
- October 2020
- September 2020
- August 2020
- July 2020
- June 2020
- May 2020
- April 2020
- March 2020
- February 2020
- January 2020
- December 2019
- November 2019
- September 2018
- October 2017
- December 2011
- August 2010