AI’s infrastructure problem isn’t tariffs, it’s unused capacity
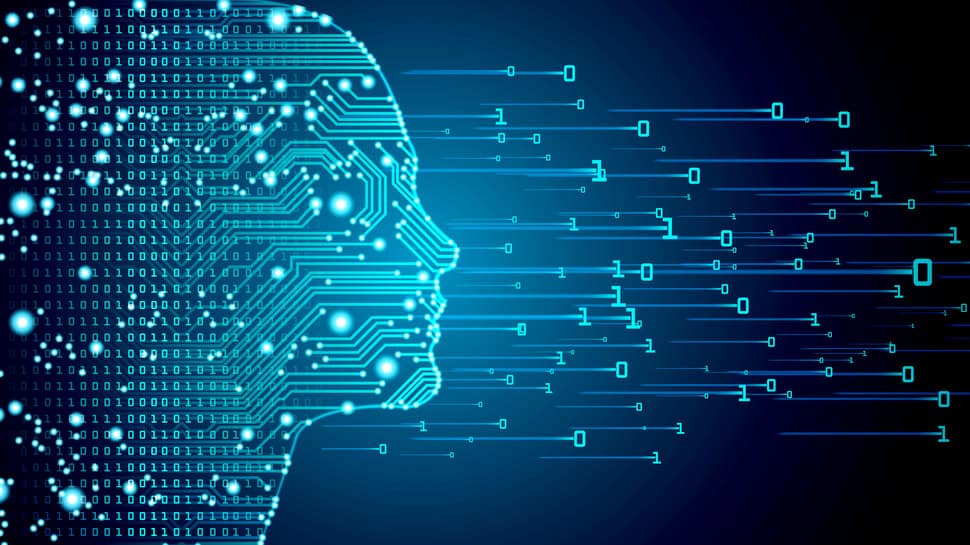
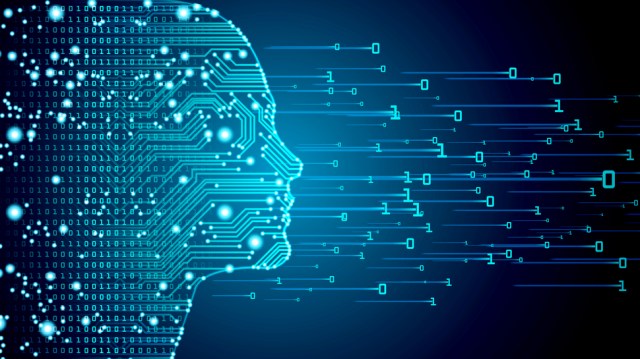
The impact that the new US administration’s sweeping global tariffs will have on building out the country’s AI infrastructure is a hot topic. This is not least because US tech giants continue to announce huge budgets for sprawling data centers, with a recent report estimating that Microsoft, Google and Meta plan to spend a combined $325 billion on new data centers.
Analysts are focusing mainly on the rising cost of steel, copper, and other precious metals needed to expand physical infrastructure, as well as the specialized semiconductor and GPU chips essential to data centers. By some estimates, construction costs for commercial projects may rise by as much as 5% under the new US global tariff regime.
While these estimates may be correct, the analysis – at least in terms of data centers – is missing a crucial element. Debates over importing steel, GPUs, servers, and networking equipment assume that meeting rising computing demand means we must continuously build. The reality, however, is that we are significantly underutilizing the computing resources we already have.
CEO of io.net.
The resource utilization problem
What we have here is a manufacturing solution being proposed for a distribution problem. Building additional facilities to meet the growing demand for AI computing not only requires enormous capital investment, but this centralized model creates vulnerabilities in terms of single points of failure and malicious actors.
Meanwhile, vast computing resources are sitting idle worldwide. Indeed, we are wasting tremendous computing power. According to the SPECpower benchmark, 20% to 60% of the power consumed by computers connected to the public cloud is idle and so unattributable to active utilization. This means that a significant amount of cloud and GPU computing power is likely being unutilized globally.
In China, the situation is markedly worse. Due to the country’s recent AI infrastructure “gold rush”, billions of dollars were spent on data centers, around 80% of which are now sitting idle, according to a report published by The MIT Technology Review.
This is because the recent release of Chinese AI LLM DeepSeek has revealed a startling truth: AI doesn’t need $30,000 GPU chips with enormous computing power to operate. Indeed, as the release of DeepSeek V3 has just shown, an AI LLM can be run on a Mac computer.
This serves to underline the enormous inefficiencies in global computing power that have persisted for years simply because we have not sought to aggregate and redistribute existing resources. Rather than building entirely new infrastructure, we can tap into reserves of existing computational power. All we need to do is rethink computing infrastructure.
Utilizing existing infrastructure
Harnessing unused computational power is the most efficient way to meet the demands of AI, and Decentralized Physical Infrastructure Networks (DePINs) offer innovative solutions. Rather than building more centralized facilities, DePIN cloud models can connect buyers with suppliers of unused computing power through a globally distributed network that is not reliant on single data centers.
One might think of this as the “Airbnb of Compute” – a way to connect those who need GPU space with those who have GPU space to spare.
This approach, which is able to utilize the world’s idle computing power, has the potential to meet the needs of businesses and individuals without spending billions of dollars on new physical infrastructure.
By taking advantage of underutilized GPU power, cloud computing DePIN platforms can offer high-performance computing at prices significantly lower than established providers like AWS and Azure, and this structural advantage makes tariff concerns largely irrelevant.
Rather than spending billions of dollars on new facilities that will – as China’s dilemma demonstrates – be underutilized, DePIN models maximize existing resources. As DeepSeek has shown, the distribution of computing power can also widen participation in AI tools, enabling small and medium enterprises to leverage machine learning technology without prohibitive costs.
Computing without constraints
Globally, we have come to realize the drawbacks of centralization. In the cloud space, by pivoting from large, centralized data centers, we can build networks that are resilient to trade wars or pandemics. These distributed systems respond to demand naturally – expanding when needed, contracting when not needed – without requiring vast new facilities.
For business leaders and policymakers, this represents an opportunity to rethink assumptions about infrastructure development. Rather than subsidizing more gigantic data centers, leaders could focus policies on incentivizing the better utilization of existing resources.
In the US especially, rather than poring over the potential increase in costs of importing more steel, copper, and computational hardware, the Stargate Infrastructure Project might focus on the vast unused capacity the country already has. And, as cost-cutting is a core focus of this administration, this approach would be fully aligned.
Perhaps of even more interest to such a conservative administration would be the savings in energy costs that such an approach would bring. Distributed computing networks typically consume less energy than large data centers and, more importantly, in a way that does not negatively impact communities and businesses in the area.
Innovation through efficiency
Most importantly, though, distributed computing networks enable faster innovation cycles by lowering barriers to access. They create new economic opportunities for resource providers previously excluded from the AI economy – and innovation and entrepreneurship are nothing if not the raison d’etre of Republican idealism.
Ultimately, current debates around tariffs and their impact on data center construction costs distract from a glaring opportunity. By embracing decentralized computing models, the world can meet growing AI demands without being constrained by infrastructure limitations. The technology exists today, and the economic case is compelling. The only remaining question is how quickly businesses and policymakers will recognize this.
We’ve featured the best IT infrastructure management service.
This article was produced as part of TechRadarPro’s Expert Insights channel where we feature the best and brightest minds in the technology industry today. The views expressed here are those of the author and are not necessarily those of TechRadarPro or Future plc. If you are interested in contributing find out more here: https://www.techradar.com/news/submit-your-story-to-techradar-pro
The impact that the new US administration’s sweeping global tariffs will have on building out the country’s AI infrastructure is a hot topic. This is not least because US tech giants continue to announce huge budgets for sprawling data centers, with a recent report estimating that Microsoft, Google and Meta…
Recent Posts
- AI’s infrastructure problem isn’t tariffs, it’s unused capacity
- Apple ‘iPhone Air’ rumor suggests a bigger screen coming in 2027
- Sightful Spacetop for Windows Review: Spatial Computing Is Here
- Gmail servers hijacked by malicious PyPI packages to spread havoc – here’s how to stay safe
- Microsoft has fixed a bug in Windows 10 that broke part of the Start menu – and the reason why this happened might annoy you
Archives
- May 2025
- April 2025
- March 2025
- February 2025
- January 2025
- December 2024
- November 2024
- October 2024
- September 2024
- August 2024
- July 2024
- June 2024
- May 2024
- April 2024
- March 2024
- February 2024
- January 2024
- December 2023
- November 2023
- October 2023
- September 2023
- August 2023
- July 2023
- June 2023
- May 2023
- April 2023
- March 2023
- February 2023
- January 2023
- December 2022
- November 2022
- October 2022
- September 2022
- August 2022
- July 2022
- June 2022
- May 2022
- April 2022
- March 2022
- February 2022
- January 2022
- December 2021
- November 2021
- October 2021
- September 2021
- August 2021
- July 2021
- June 2021
- May 2021
- April 2021
- March 2021
- February 2021
- January 2021
- December 2020
- November 2020
- October 2020
- September 2020
- August 2020
- July 2020
- June 2020
- May 2020
- April 2020
- March 2020
- February 2020
- January 2020
- December 2019
- November 2019
- September 2018
- October 2017
- December 2011
- August 2010