OpenAI spent $80M to $100M training GPT-4; Chinese firm claims it trained its rival AI model for $3 million using just 2,000 GPUs
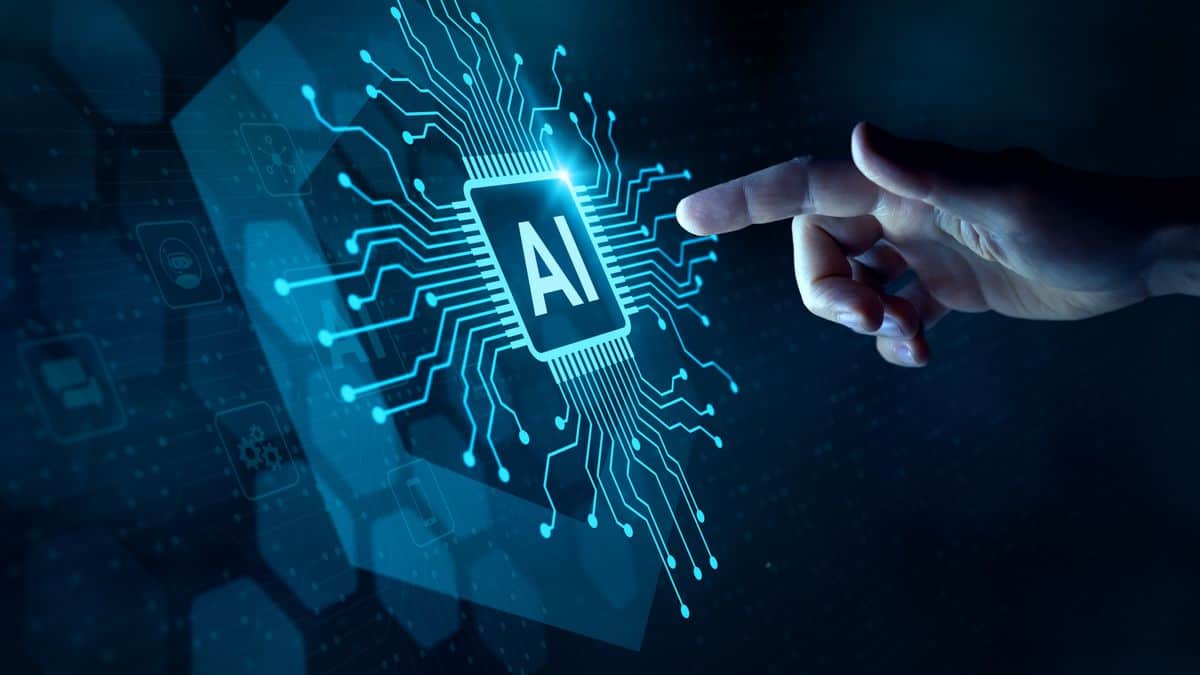
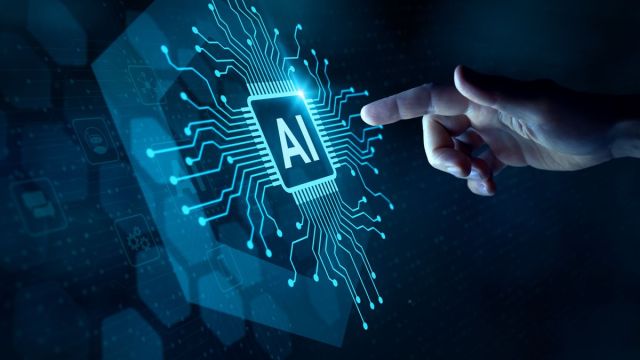
- 01.ai trained an AI model for $3 million using 2000 unnamed GPUS
- “Efficient engineering” allows 01.ai to compete globally, company claims
- 01.ai reduced inference costs to 10 cents per million tokens
Tech companies in China face a number of challenges due to the American export ban, which restricts access to advanced hardware from US manufacturers.
This includes cutting-edge GPUs from Nvidia, critical for training large-scale AI models, forcing Chinese firms to rely on older or less efficient alternatives, making it difficult to compete globally in the rapidly evolving AI industry.
However, as we’ve seen time and again, these seemingly insurmountable challenges are increasingly being overcome through innovative solutions and Chinese ingenuity. Kai-Fu Lee, founder and CEO of 01.ai, recently revealed that his team successfully trained its high-performing model, Yi-Lightning, with a budget of just $3 million and 2,000 GPUs. In comparison, OpenAI reportedly spent $80-$100 million to train GPT-4 and is rumored to have allocated up to $1 billion for GPT-5.
Making inference fast too
“The thing that shocks my friends in the Silicon Valley is not just our performance, but that we trained the model with only $3 million,” Lee said (via @tsarnick).
“We believe in scaling law, but when you do excellent detailed engineering, it is not the case you have to spend a billion dollars to train a great model. As a company in China, first, we have limited access to GPUs due to the US regulations, and secondly, Chinese companies are not valued what the American companies are. So when we have less money and difficulty to get GPUs, I truly believe that necessity is the mother of invention.”
Lee explained the company’s innovations include reducing computational bottlenecks, developing multi-layer caching, and designing a specialized inference engine. These advancements, he claims, result in more efficient memory usage and optimized training processes.
“When we only have 2,000 GPUs, the team has to figure out how to use it,” Kai-Fu Lee said, without disclosing the type of GPUs used. “I, as the CEO, have to figure out how to prioritize it, and then not only do we have to make training fast, we have to make inference fast… The bottom line is our inference cost is 10 cents per million tokens.”
Sign up to the TechRadar Pro newsletter to get all the top news, opinion, features and guidance your business needs to succeed!
For context, that’s about 1/30th of the typical rate charged by comparable models, highlighting the efficiency of 01.ai’s approach.
Some people may be skeptical about the claims that you can train an AI model with limited resources and “excellent engineering”, but according to UC Berkeley’s LMSIS, Yi-Lightning is ranked sixth globally in performance, suggesting that however it has done it, 01.ai has indeed found a way to be competitive with a minuscule budget and limited GPU access.
You might also like
01.ai trained an AI model for $3 million using 2000 unnamed GPUS “Efficient engineering” allows 01.ai to compete globally, company claims 01.ai reduced inference costs to 10 cents per million tokens Tech companies in China face a number of challenges due to the American export ban, which restricts access to…
Recent Posts
- Razer’s new Blade 18 offers Nvidia RTX 50-series GPUs and a dual mode display
- I tried adding audio to videos in Dream Machine, and Sora’s silence sounds deafening in comparison
- Sandisk quietly introduced an 8TB version of its popular portable SSD, and I just hope they solved its previous big data corruption issue
- iPhones are briefly changing ‘racist’ to ‘Trump’ due to an iOS dictation issue
- We finally know who’s legally running DOGE
Archives
- February 2025
- January 2025
- December 2024
- November 2024
- October 2024
- September 2024
- August 2024
- July 2024
- June 2024
- May 2024
- April 2024
- March 2024
- February 2024
- January 2024
- December 2023
- November 2023
- October 2023
- September 2023
- August 2023
- July 2023
- June 2023
- May 2023
- April 2023
- March 2023
- February 2023
- January 2023
- December 2022
- November 2022
- October 2022
- September 2022
- August 2022
- July 2022
- June 2022
- May 2022
- April 2022
- March 2022
- February 2022
- January 2022
- December 2021
- November 2021
- October 2021
- September 2021
- August 2021
- July 2021
- June 2021
- May 2021
- April 2021
- March 2021
- February 2021
- January 2021
- December 2020
- November 2020
- October 2020
- September 2020
- August 2020
- July 2020
- June 2020
- May 2020
- April 2020
- March 2020
- February 2020
- January 2020
- December 2019
- November 2019
- September 2018
- October 2017
- December 2011
- August 2010